Researchers from the University of Science and Technology of China (USTC), led by Prof. LIU Qi, in collaboration with Harvard Medical School’s Marinka Zitnik lab, have developed a novel deep generative algorithm, PocketGen. This algorithm, based on graph representation learning and protein language models, efficiently generates protein pocket sequences and spatial structures for binding small molecules. The study was published in Nature Machine Intelligence.
Functional protein design, particularly for proteins binding to small molecules such as enzymes and biosensors, is crucial for drug discovery and biomedical applications. Traditional methods based on energy optimization and template matching are time-consuming and yield low success rates. Meanwhile, deep learning models face challenges in modeling complex molecular-protein interactions and capturing sequence-structure dependencies. PocketGen addresses these issues, offering a high-efficiency and high-accuracy solution that adheres to physicochemical principles.
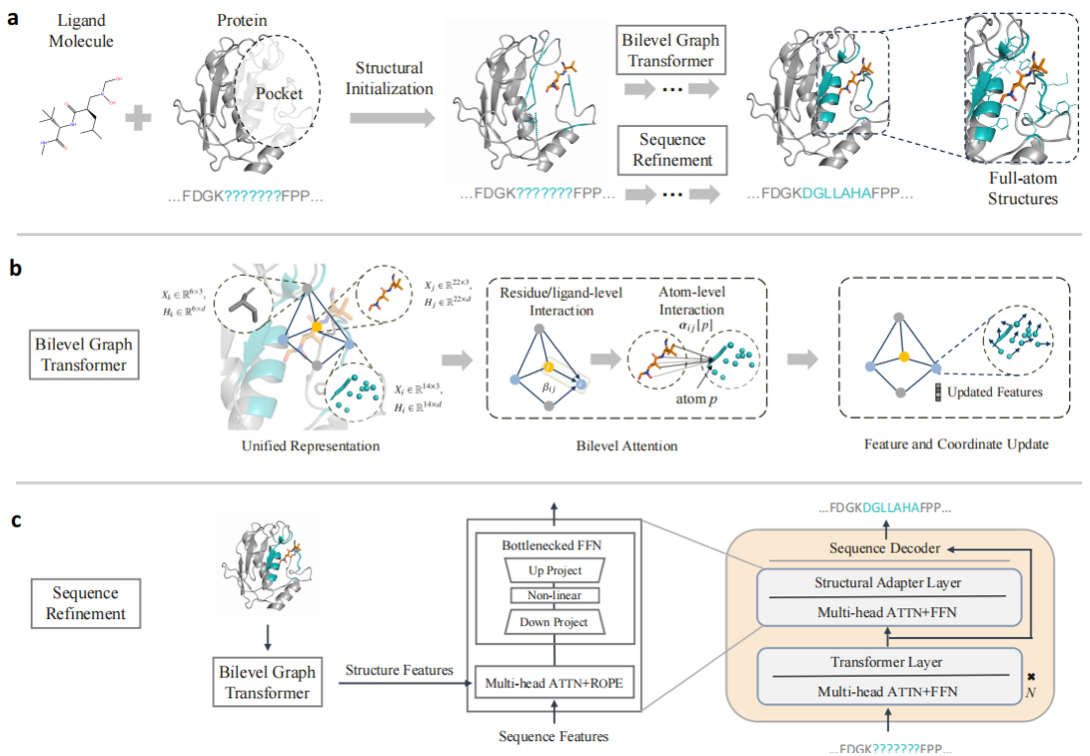
(a) Protein sequence-structure co-design with PocketGen; (b) Dual-layer graph Transformer encoder; (c) Pre-trained protein language model for sequence prediction and efficient fine-tuning. (Image from USTC)
PocketGen builds on previous works FAIR and PocketFlow and consists of two core components. First is a dual-layer graph Transformer encoder inspired by proteins’ hierarchical structures. This module is designed to learn different fine-grained interaction information and to update the representations and spatial coordinates of amino acids and atoms accordingly. The second part is a pre-trained protein language model, as illustrated in figure, where PocketGen efficiently fine-tunes the ESM2 model to assist in amino acid sequence prediction. By selectively adapting certain parameters, PocketGen enhances sequence-structure consistency through cross-attention mechanisms.
Experimental results demonstrated that PocketGen significantly outperforms traditional methods in affinity, structural plausibility, and computational efficiency, achieving over a 10-fold improvement in speed. Further, in validation tasks such as protein pocket design for small molecules like fentanyl and ibuprofen, the effectiveness of PocketGen was confirmed through comparisons with state-of-the-art generative models, including RFDiffusion and RFDiffusionAA, developed by Nobel Laureate David Baker’s lab.
Additionally, the attention matrices generated by PocketGen were compared with results from first-principle-based force field simulations, demonstrating that the deep learning-based PocketGen model exhibits good interpretability.
This work advances the application of deep generative models in functional protein design, laying a foundation for further biological experimentation and providing valuable insights into protein design principles. It also highlights the potential of AI to address critical challenges in drug discovery and bioengineering.
Paper link: https://www.nature.com/articles/s42256-024-00920-9
(Written by CHEN Yehong, Edited by WU Yuyang, USTC News Center)