How can Artificial Intelligence (AI) help accelerate scientific discovery based on vast amounts of experimental data? A new study by Prof. LI Weixue’s team from the University of Science and Technology of China (USTC) of the Chinese Academy of Sciences shows how this can be achieved in heterogonous catalysis. The results were recently published in Science.
By integrating interpretable AI with experimental data, domain knowledge, and first-principles simulations, the researchers established a general theory of metal-support interaction (MSI), which is one of the most important pillars in catalysis.
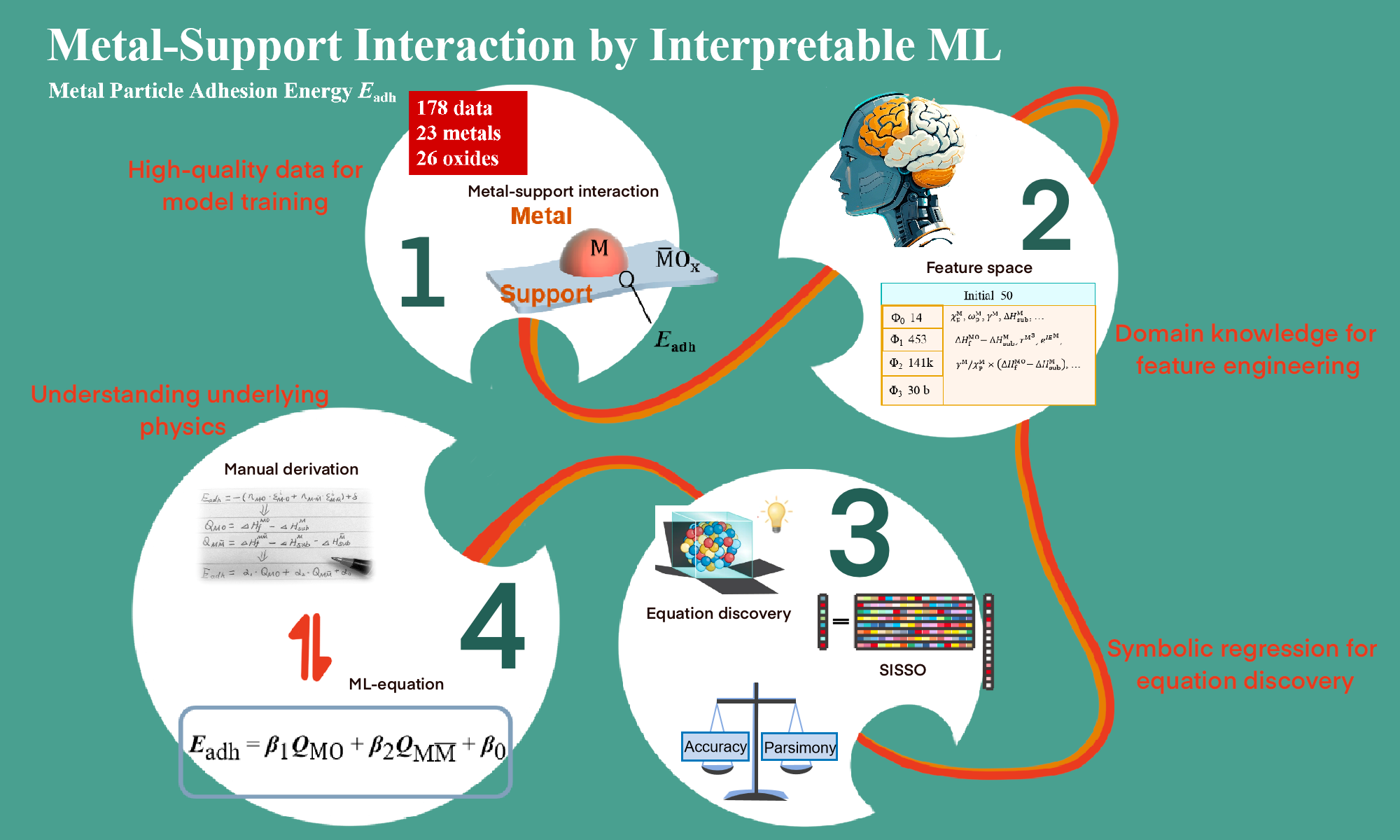
Metal-Support Interaction by Interpretable ML (Image by USTC)
Supported metal catalysts have been widely used for petrochemical refining and industrial chemical manufacturing as well as environmental control systems such as exhaust catalysts. MSI helps stabilize dispersed catalysts and impacts interfacial processes such as charge transfer, chemical composition, perimeter sites, particle morphology, and suboxide encapsulation. Modulation of MSIs is thus one of the few strategies for enhancing catalyst performance.
For example, strong MSIs, which were originally used to describe the encapsulation of supported metal nanoparticles by suboxide layers at elevated temperatures—a discovery made in 1978—have received much attention recently and have been thought of as the origin of many prominent interfacial processes. However, fundamental questions remain about the nature of (strong) MSIs and their influence on interfacial processes in general and encapsulation in particular, due to the intricate interfaces and complex processes involved.
In this study, Prof. LI's team made a breakthrough on this issue. LI envisioned that a concise formula for accurately describing and predicting the strength of MSI should exist. The team then compiled consistent experimental data from previous seminal research covering 25 metals and 27 oxides. Using advanced interpretable machine learning algorithms, combined with domain knowledge and theoretical derivation, the team identified a formula to predict MSI from basic and easy-to-obtain material parameters.
This concise formula revealed that MSI strength is the sum of the metal-metal and metal-oxygen interactions across the interface. The metal-metal interaction, a surprisingly novel quantity but not recognized before, contributes significantly to MSI relative to the widely acknowledged metal-oxygen interaction. The proposed formula exhibits remarkable universality. It can be applied not only to oxide-supported metal nanoparticle catalysts but also to metal single-atom catalysts and metal-supported oxide catalysts. This finding highlights that metal-metal interaction is the key factor in support effects, opening a new avenue for understanding and engineering such support.
Further large-scale molecular dynamic simulations based on neural-network potentials revealed that this metal-metal interaction also determines kinetic rates of oxide encapsulation over metal catalysts and the proportion of metal-metal bonds at the encapsulation interface. Based on these findings, the team proposed a principle of strong metal-metal interaction describing the occurrence of encapsulation, which not only explains nearly all observed encapsulation phenomena but also predicts new systems yet to be discovered. The principle of strong metal-metal interaction in encapsulation can be extrapolated to the encapsulation of other metal compound supports, providing theoretical guidance on interface design and engineering.
“This achievement resolves a major fundamental scientific challenge in heterogeneous catalysis and provides immense potential for the design of efficient supported catalysts,” said Academician LI Yadong from Tsinghua University.
“This breakthrough is expected to accelerate the discovery of new catalytic materials and reactions, advancing the field of catalysis in energy, environment, and material science, thereby contributing to the sustainable development of society,” said Prof. LI Weixue.
This study shows the potential of integrating interpretable AI algorithms with domain knowledge to build mathematical models and extract scientific principles based on vast amounts of historical experimental data. In this way, the study offers a novel perspective on scientific discovery in chemistry in the age of “AI for Science.”
Paper link: http://science.org/doi/10.1126/science.adp6034
(Written by WANG Hanzhi, Edited by ZHANG Yihang, ZHAO Zheqian, USTC News Center)